Artificial intelligence (AI) allows utilities to address pressing challenges, including aging infrastructure, workforce turnover and operational complexity. ScottMadden views AI as an umbrella term, encompassing a range of transformative technologies, including generative AI (e.g., chatbots, automated reporting), machine learning (predictive maintenance, anomaly detection), computer vision (equipment inspections, corrosion detection) and deep learning (complex data modeling and real-time decision-making). Utilities can move beyond experimentation to achieve measurable operational improvements while laying the foundation for sustained innovation by adopting a structured approach to selecting, prototyping and scaling AI use cases.
Insights from the field: Industry poll results
At the EPRI AI and Digital Transformation Summit in January 2025, audience polls revealed key insights into AI adoption in utilities:
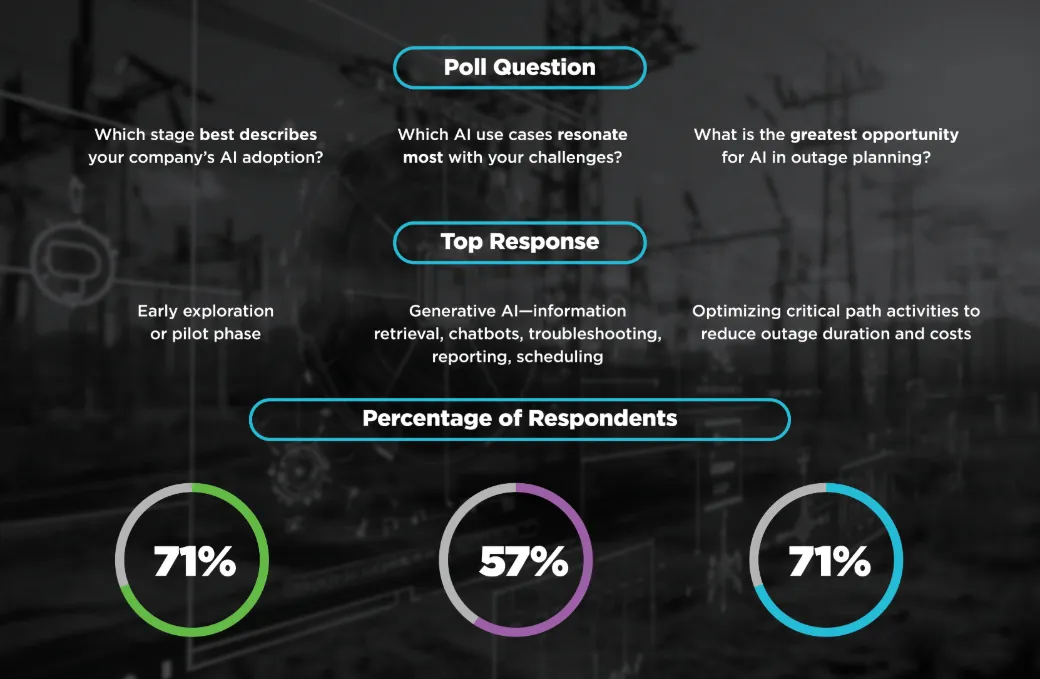
The results highlight that most utilities are still piloting AI, focusing on generative AI applications and outage planning optimization as practical starting points. This reflects a need for early successes in targeted areas to build momentum for broader adoption.
Adapting strategies based on insights
To translate interest into action, utilities must focus on high-value, practical use cases that balance feasibility with impact. Generative AI applications—such as automating reporting or scheduling—are especially appealing for their ability to streamline repetitive tasks. Similarly, optimizing outage planning remains a high-priority use case, leveraging analytics and historical outage data to minimize costs and improve scheduling. By targeting these areas, utilities can achieve early wins while laying the groundwork for long-term AI integration.
Overcoming barriers with structured use case selection
AI adoption requires a methodical approach to ensure alignment with organizational goals and address technical challenges. Key steps include:
- Identify high-impact use cases: Focus on areas with measurable value, such as predictive maintenance and compliance automation.
- Prototyping and iteration: Develop small-scale prototypes to test feasibility and build confidence. Synthetic data—artificially generated datasets that mimic real-world conditions—can accelerate validation while overcoming data access challenges.
- Assess real data readiness in parallel: In tandem with using synthetic data, utilities should evaluate the quality, completeness and structure of their real-world data to prepare for long-term AI implementation.
- Engage stakeholders: Cross-functional collaboration ensures solutions address operational needs and gain organizational buy-in.
This structured process minimizes risks while positioning utilities to effectively scale their AI initiatives.
Experimentation as a driver of AI adoption
Experimentation plays a crucial role in advancing AI adoption by enabling utilities to explore its potential in controlled environments. Examples include:
- Workweek schedule optimization: AI tools analyze resource allocation, flag inefficiencies and balance workloads.
- Generative AI for report automation: Automating engineering, compliance and regulatory reporting reduces manual workloads and improves accuracy.
These initiatives help build organizational confidence with low-risk processes and prepare utilities for broader integration of AI solutions.
Lessons learned from early deployments
Insights from ScottMadden’s recent projects highlight key lessons:
- Start small and scale: Focus on narrowly defined projects, like predictive maintenance pilots, to deliver quick wins and build momentum.
- Address data challenges proactively: Data quality is critical but addressing it can take time. While synthetic data accelerates validation, it is essential to assess and prepare real-world data in parallel to ensure prototypes can eventually transition into production environments.
- Collaborate across functions: Cross-functional collaboration ensures AI solutions align with both operational realities and strategic goals.
- Design for scalability: Prototypes should be adaptable, enabling future scaling to other sites or applications.
AI in action: Key use cases
AI’s potential spans both transmission and distribution (T&D) and power generation. Here are some high-impact applications:
Predictive maintenance:
- T&D: AI-driven computer vision analyzes drone images to detect corrosion or structural issues on transmission towers.
- Generation: Predictive tools identify turbine bearing failures, boiler overheating and SCRAM (emergency reactor shutdown) risks in nuclear facilities, reducing downtime and improving safety.
Operational efficiency:
- T&D: AI improves grid balancing by analyzing real-time supply and demand data and optimizing energy flows.
- Generation: AI manages hydroelectric pump operations and optimizes combustion in gas plants, enhancing energy output and efficiency.
Compliance and corrective action:
- Corrective action program automation: AI streamlines investigation workflows by categorizing incidents, recommending resolutions and generating reports, saving time and improving consistency.
OT cybersecurity:
- Prioritizing vulnerabilities: AI identifies high-risk cybersecurity threats across operational technology (OT) systems, helping utilities allocate resources to critical issues.
- Tuning behavioral alerts: By combining security and physical sensor data, AI improves the accuracy of anomaly detection, reducing false positives, which improves efficiency and enables faster response to threats.
These examples demonstrate AI’s versatility in enhancing reliability, efficiency and safety. For further details, see ScottMadden’s articles on AI Use Cases in Power Generation and AI Use Cases in Transmission & Distribution.
A Roadmap for scaling AI adoption
A phased approach enables utilities to scale AI sustainably:
- Pilot and prove value (0–6 months): Develop small-scale prototypes, using synthetic data to validate high-potential use cases while assessing real-world data readiness.
- Deploy and expand (6–18 months): Scale successful pilots across multiple sites and refine tools for broader application.
- Integrate and optimize (18–36 months): Embed AI solutions into enterprise systems for real-time data insights and operational decision-making.
- Scale and transform (36+ months): Drive enterprise-wide adoption, leveraging AI to optimize assets and enhance workforce capabilities.
Call to action: Start small. think big
Utilities are at a pivotal moment in their AI journeys. By focusing on practical use cases, leveraging synthetic data to address challenges, and experimenting with prototypes, they can help their teams meaningfully engage and learn the potential of AI and unlock its significant operational benefits.